
2023 was a year of groundbreaking developments in AI.
The underlying large language models (LLMs) underwent rapid iterations, and AI rapidly unfolded on a global scale. From ChatGPT, the fastest-growing application in user base in history, to AutoGPT, the open-source project with the quickest increase in stars on GitHub; from the dazzling debut of GPT-4 to Meta's release of Llama, democratizing and open-sourcing the acceleration of LLMs, and Google Gemini's emergence with multimodal capabilities—businesses transitioned from being unprepared to actively considering AI strategies and experimenting with the implementation of LLMs across various industries.
The development of LLMs are represented by two paths: the closed-source models, with OpenAI's GPT as a flagship, and the open-source models, led by the Llama series.
In the closed-source domain, OpenAI represents the cutting edge of the industry. Each release provides forward-looking guidance for the industry's development and commercialization.
The milestone of GPT-4's release marked a significant point in 2023, with several competitors subsequently introducing LLMs almost matching the performance of GPT-4.
Towards the end of the year, the release of Google Gemini marked the true beginning of genuinely multimodal models.
In the open-source field, Meta's Llama2 currently boasts the best performance and highest usage, although its performance is only equivalent to GPT-3.5.
The release of Mistral AI's MoE model towards the end of the year brought some hope for open source catching up to GPT-4.
The open-source ecosystem thrived throughout the year: by November 2023, over 300,000 models were released on HuggingFace, more than 200,000 developers contributed to AI projects on GitHub, and the user base on AI-related Discord channels exceeded 18 million.
Open Source vs. Closed Source LLM Gap
We believe that in the realm of LLMs, these two paths of open source and closed source will coexist for an extended period, serving different users and scenarios.
Closed-source models will continue to lead in performance until they encounter bottlenecks, and the gap is likely to widen. Several reasons contribute to this:
Core Resources: The core resources influencing LLMs are computing power and data, where open-source models are at a disadvantage. OpenAI's efforts in developer ecosystems and application platforms enable it to occupy more users and data, coupled with financial support, ensuring its ongoing advantage.
Developer Contributions: There is a fundamental difference between open-source models and open-source software. Developers' contributions to the former are limited, lacking the continuous improvement seen in basic software through code contributions. This absence of the ecosystem effect of "many hands make light work" hinders the development of open-source models.
Costs and Support: OpenAI's decreasing costs and the support of enterprise versions will further consume the market space for open-source models. While open-source models have advantages in terms of being "cheap" and "secure," the release of GPT-4 Turbo at the end of last year showed a rapid decrease in OpenAI's costs. As technology advances and scales, costs are expected to further decrease. From the perspective of data security, OpenAI collaborates with Microsoft Azure to ensure the data security of enterprise-level customers.

The future of open-source models may lie in small-scale and customization. (Cost is crucial when deploying privately within enterprises.)
Small-scale models are more suitable for 2B scenarios, with Llama2 7B model showing the best cost-effectiveness and broadest applications in enterprise usage scenarios.
Closed-source models serve as universal infrastructure, posing challenges for customization. In specific fields, using open-source models may offer more flexibility.
Startups might use OpenAI for prototype design. Once validated, they train a small model using open-source models to achieve similar performance to GPT at lower costs in specific tasks.
In March 2023, Microsoft released Office Copilot, signaling the emergence of a fundamental framework for building applications based on LLMs, which later evolved into Agents. In less than a year, Agents platform has quickly gained industry consensus, becoming the fundamental paradigm for hosting applications built on LLMs.
OpenAI Trends & AI Agents
Looking deeper at OpenAI, there are two clear strategic trends:
- continually enhancing the performance of foundational LLMs, aiming for Artificial General Intelligence (AGI);
- focusing on building the capabilities and systems around Agents, continuously enriching its own Agents platform. Starting with the Plugin Platform in early 2023, Function Calling in June, Assistant API in November, and GPTs Store and GPT mentions released in January of this year, OpenAI has been consistently experimenting and exploring in this direction. Currently, they have progressively built a comprehensive and user-friendly Agents platform, facilitating developers in constructing Agents.
With the rapid improvement of underlying LLM capabilities and the gradual refinement of the AI Agents platform, the cost of building agents has significantly decreased and is expected to continue doing so. This provides a solid foundation for companies venturing into agents development.
The core capability of domain-specific agents lies in mastering domain knowledge and having a profound understanding of domain-specific business features. The "technical costs" beyond this aspect have been considerably reduced by LLMs. In the past, industries were vertically divided, and an AI startup had to cover everything from the foundational level to applications, which is not sustainable for most startups. In the future, the low startup costs will lead to an "Agents" explosion, creating substantial economic value, driven by the exponential reduction in the cost enabled by AI.
In the future, the domain of AI Agents will undoubtedly be a diverse market, well-suited for teams with creativity and specific domain knowledge. While the entry barrier is not high, the upper limit is considerable. Well-designed, domain-specific agents can take over a significant portion of tasks, potentially revolutionizing how certain industries operate.
Retrieval-Augmented Generation (RAG) and Optimization Techniques for Large Models
Despite the powerful capabilities of LLMs, Agents built upon them face significant challenges in real-world applications, such as hallucinations, slow knowledge updates, and a lack of transparency in answers. To address these issues, Retrieval-Augmented Generation (RAG) has emerged and quickly gained development.
Retrieval-Augmented Generation (RAG) is a retrieval-augmented generation technology that addresses the above challenges by externally retrieving relevant information and guiding the generation process using this information. In doing so, RAG effectively mitigates hallucination issues, significantly enhancing the accuracy and relevance of generated content. Simultaneously, it tackles the slow knowledge update problem, strengthening the traceability of content generation, making LLMs more practical and trustworthy in real-world applications.
In addition to RAG, optimization techniques for large models include Prompt Engineering and Supervised Fine-tuning (SFT), each with its own characteristics and suitable scenarios. RAG and SFT complement each other, and their combined use may yield optimal performance.
Year in Review: 2023 Milestones in the Era of Large Language Models
On November 30, 2022, OpenAI released ChatGPT, causing a sensation in the industry. By the end of January 2023, OpenAI announced that its monthly active users had surpassed 100 million, making it the fastest-growing application in user numbers in history. This marked the beginning of an extraordinary year for AI in 2023.
Let's summarize the milestones that occurred throughout this year.
February 24, 2023: Meta releases the LLaMA series large models, one-tenth the scale of GPT-3 but surpassing GPT-3 in most benchmark tests.
March 15, 2023: OpenAI officially releases GPT-4. Compared to GPT-3.5, GPT-4 demonstrates near or at human-level performance in many real-world scenarios and introduces "multimodal" capabilities, accepting both images and text as inputs.
March 16, 2023: Microsoft releases Microsoft 365 Copilot, introducing an application development paradigm based on Large Language Models.
March 24, 2023: OpenAI introduces Plugins, connecting ChatGPT to third-party applications, enabling interaction with external APIs.
April 2023: Autonomous Agents gain popularity rapidly, AutoGPT became the fastest-growing project in GitHub history.
May 10, 2023: Google releases the large model PaLM2, benchmarked against GPT-4.
June 2023: OpenAI introduces the Function Calling feature, allowing users to describe functions and enable the model to intelligently choose outputs in JSON format.
July 12, 2023: Anthropic releases the large model Claude2, supporting long-text input-output—each prompt can input up to 100,000 tokens.
July 18, 2023: Meta releases the open-source large model Llama2, showing performance close to GPT-3.5 in various benchmark tests.
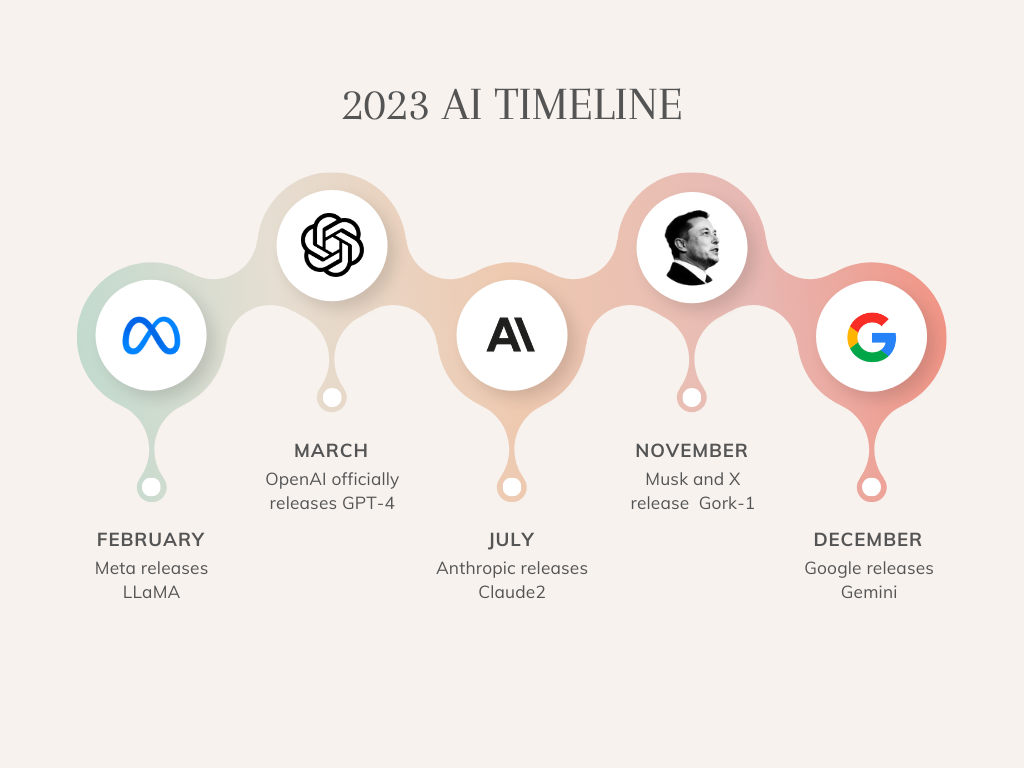
November 5, 2023: xAI, a new AI company under Elon Musk, releases its first large model, Gork-1, just four months after the company's establishment. The model's performance is equivalent to GPT-3.5, with a unique advantage of being trainable based on real human data from the xAI platform.
November 6, 2023: OpenAI DevDay introduces Assistants API.
November 7, 2023: OpenAI releases GPT-4 Turbo, making six significant enhancements and significantly reducing the cost compared to GPT-4—two-thirds lower in usage costs and faster speed.
December 7, 2023: Google releases Gemini. It is a multimodal large model, meaning it can understand, manipulate, and combine different types of information, including text, code, audio, images, and videos.
December 8, 2023: Mistral AI announces the open-source sparse mixture of experts model (SMoE), Mistral 8x7B. It matches or even surpasses Llama2 (70B) and GPT-3.5 in test scores. MoE decomposes large models into multiple "expert" submodules, each responsible for handling a specific aspect or subset of input data.
January 11, 2024: OpenAI launches GPT Store, allowing users to incorporate features like RAG on the GPT-4 model, creating GPTs.
January 27, 2024: OpenAI introduces GPT Mentions, an extension of GPT Store. Users can efficiently call other GPTs within GPT Store, presenting a more efficient multi-agent calling approach.
LLM & AI Trends for 2024
Looking ahead to 2024, we believe the following two aspects will bring significant breakthroughs for AI.
Multimodal Capabilities
If 2023 was the "year of large language models," then 2024 is likely to be the "year of multimodal large models." The upcoming releases of OpenAI GPT-4.5 and GPT-5 will expand the battleground of large models into the realm of multimodality. With the introduction of visual modalities, a new level of intelligence in large models could finally arrive:
Visual modalities possess a stronger abstraction capability for information in certain aspects, with a much larger bandwidth than text. A picture is worth a thousand words. With visual modalities, the bandwidth for interaction between large models and humans is significantly increased, allowing for more cost-effective and efficient processing of vast amounts of information.
Numerous time-series information will naturally be conveyed to large models, and describing such information in language would entail a substantial workload. This will greatly enhance the large models' cognitive understanding of the physical world, thereby substantially improving their reasoning capabilities.
The advancement brought about by multimodal capabilities in large models will accelerate the pace of AI implementation across various industries.
Personalized Agents
OpenAI GPTs has unveiled a crucial direction for the future of Agents: Personalized Agents. This concept involves Agents continuously learning based on individual user data to achieve personalization.
In the future, there might be various personalized AI agents becoming integral parts of both personal and professional life.
Behind the development of AI technology, a thread has been influencing the overall direction of the entire industry: the attitudes towards AI. Currently, there are two movements: E/acc (Effective Accelerationism) and EA (Effective Altruism).
E/acc Movement (Effective Accelerationism): Proponents, such as Sam Altman and Gary Tan, advocates leveraging the power of capital and technology to rapidly drive AI innovation, actively promoting the commercialization of large models. The E/acc faction believes that new technologies will inevitably bring both benefits and drawbacks to human society, and these are unavoidable challenges humanity must face.
EA Movement (Effective Altruism): Proponents, such as Ilya Sutskever and Geoffrey Hinton, argues that the current focus should be on the risks of Artificial General Intelligence (AGI), particularly safety and interpretability. They are concerned that once general artificial intelligence emerges, the situation might surpass human control. Therefore, they emphasize a cautious approach to the development of AGI.
- Streamline Your Workflow with Leiga
- Effortlessly automate tasks
- Boost productivity with AI insights
- Free forever — elevate project management